Artificial Intelligence In Autonomous Vehicles
Artificial intelligence (AI) is playing an ever-increasing role in the automotive industry and is considering the key to autonomy for self-driving cars. But why is that? The human being is remarkable in that it can drive vehicles at high speed and in urban areas with many things happening on the road and to the side – and yet a person can, usually, manage all these visual and aural cues without causing an accident.
These things are very challenging for a computer. For all these inputs, such as traffic lights, pedestrians, other cars on the road, roadworks, and challenges such as rain, fog and snow, the vehicle needs to replicate the ability of the human brain to sense what is happening. It needs to anticipate and predict what will happen next, and then recommend a course of action. The AI has to decide on whether to slow the car down by applying the brake or to drive carefully to avoid an obstacle.
Artificial intelligence doesn’t work in isolation. AI advances rely on a lot of hardware, optimised for autonomous, self-driving vehicles. In turn, that hardware relies on autonomous software. The three, together, deliver truly autonomous vehicles.
As the growth in capability of trained neural networks has increased there have been many breakthroughs for artificial intelligence. The self-driving car is, therefore, becoming a reality.
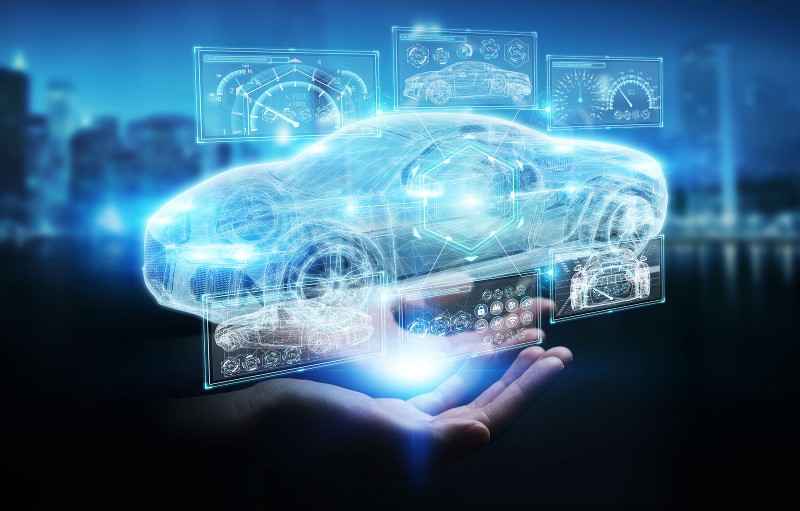
The car will soon be a data centre on wheels
AI is now a fundamental part of our world. Though we may not be aware of it, artificial intelligence is involved whenever we search for a word on the web, when we make a financial transaction or when we have a filter applied to a picture on our smartphones.
Through neural networks, AI has been putting the smart in smartphone, and now it’s putting the smartness in smart cars. Car manufacturers and start-ups focusing on self-driving cars are looking to apply AI to enable us to reach the ultimate goal of creating fully autonomous vehicles – that is, cars that can drive themselves.
This requires a mastery of computer vision, for the computer inside the car to understand the world as we do, but to enable the car to react faster and more effectively than a human ever could to dangerous situations.
This is where the power of neural networks comes in. A key feature of machine learning, neural networks mimic the design of the human brain to allow machines to recognise patterns, and objects the same way we do – and ideally even faster and more efficiently.
The concept of the neural network has been around for a long time, but they require considerable compute power to be effective. This is why they are now coming into their own. Thanks to the relentless increase in processor power, they can now be run faster and more efficiently, on cost and low-power hardware. Originally running on CPUs, they initially received a massive speed boost thanks to the power of graphics processing units (GPU), which through their inherently massively parallel compute engines, proved ideal for running compute tasks.
However, as the name suggests, dedicated neural network accelerators are designed specifically for these tasks. As such, they are far more power and area efficient than even GPUs, making them orders of magnitude more effective at running neural networks for inferencing on the edge.
The self-driving car is becoming a reality.
This efficiency means it’s now possible to run the required AI models directly on an edge device, (such as in a vehicle), without relying on cloud computing, which would entail the round-trip time of contacting a data centre. It means we now get the power and predictability of running neural networks locally, something that only a few years ago would have been the exclusive domain of the data centre – but now is possible on the data centre on wheels. This is enabling AI to transform the automotive sector.
By running neural networks locally, all benefits of the autonomous car, from functional safety to multi-core flexibility, can run quicker and faster, and all with low power consumption.
Related Articles
What Software Is Needed For Autonomous Vehicles?
Related Posts
Learning from the best: How nature can inspire AI research
Imagination China sees 2020 out in award-winning style with IMG Series 4 NNA
Running LSTM neural networks on an Imagination NNA
Imagination and Humanising Autonomy Part 1: The path to safer roads
Imagination and Humanising Autonomy Part 2: The humans behind the autonomy